Introduction
Stock forecasting has always been a crucial aspect of investing, as it helps investors predict the future performance of a company’s stock and make informed decisions. Traditionally, stock forecasting relied on fundamental and technical analysis, but the advent of artificial intelligence (AI) has brought significant improvements to this field. AI stock forecasting, such as the one provided by FinBrain Technologies, has the potential to revolutionize the way investors approach the stock market.
Evolution of stock forecasting methods
Traditional methods of stock forecasting included fundamental analysis, which focuses on a company’s financial health and overall market conditions, and technical analysis, which looks at past stock price movements to predict future trends. However, these methods have limitations, such as being time-consuming, subjective, and not always accurate.
With the rise of AI and machine learning, new techniques have emerged that can process large amounts of data faster and more accurately than humans can. These AI-based methods include machine learning, neural networks, and deep learning, and have proven to be more efficient and reliable than traditional methods in many cases.
AI techniques used in stock forecasting
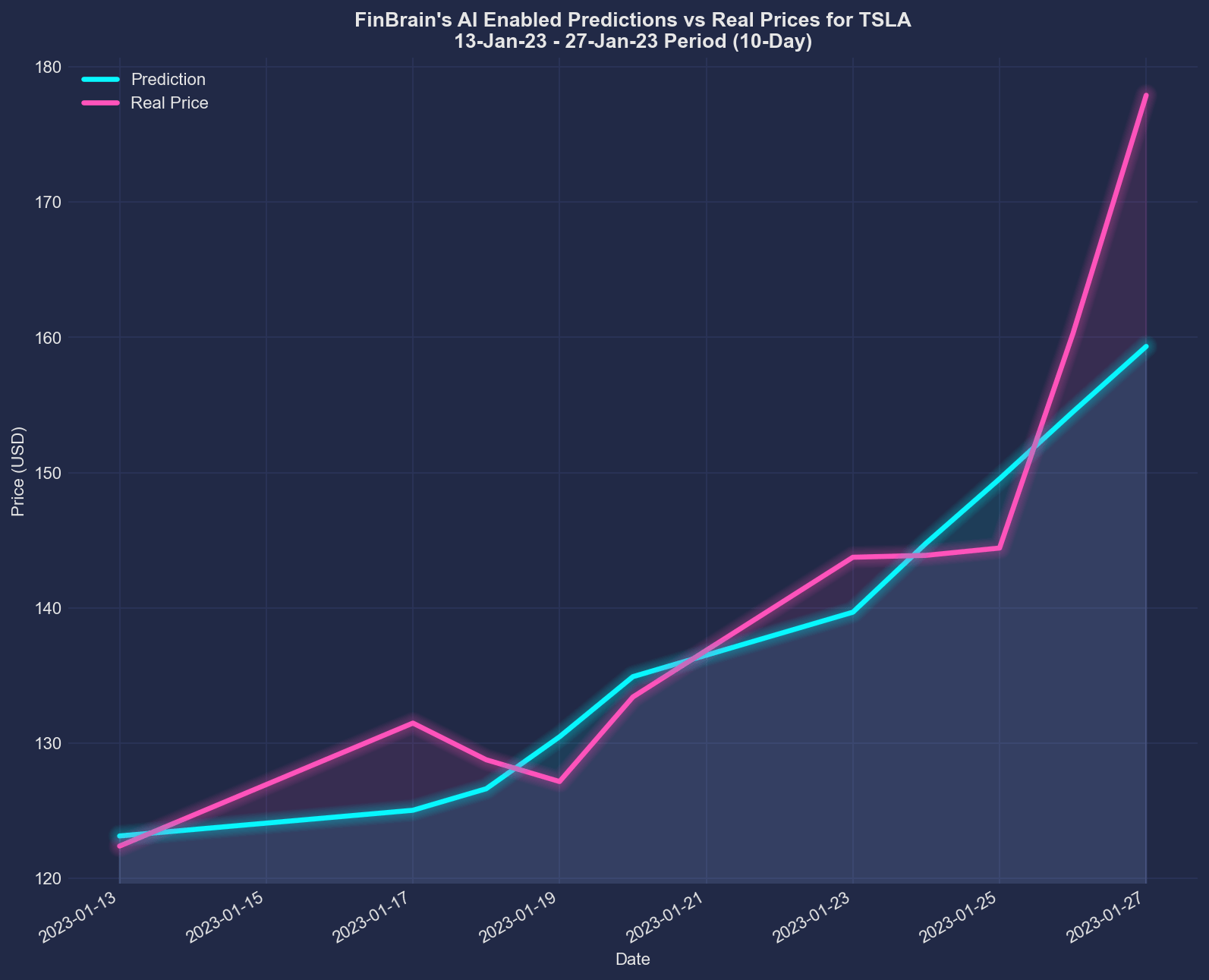
AI stock forecasting employs various algorithms and techniques to analyze financial data and predict stock prices. Here is more information about some of the most common techniques:
Machine learning algorithms
- Linear regression: Linear regression is a simple machine learning algorithm that models the relationship between a dependent variable (stock price) and one or more independent variables (such as market factors or financial metrics). It identifies a straight line that best fits the data points, minimizing the differences between the predicted and actual values.
- Support vector machines: Support vector machines (SVMs) are a powerful classification and regression algorithm that can be applied to stock forecasting. SVMs work by finding the optimal hyperplane that separates data points into different categories (e.g., price increase or decrease) with the largest margin possible. This technique can handle nonlinear relationships and is less prone to overfitting.
- Random forests: Random forests are an ensemble learning method that constructs multiple decision trees and combines their outputs to make a final prediction. This approach offers better accuracy and robustness compared to single decision trees by reducing the risk of overfitting and mitigating the impact of individual tree errors.
Neural networks
- Feedforward neural networks: Feedforward neural networks consist of multiple layers of interconnected neurons, with each layer processing the input data and passing the results to the next layer. These networks can model complex relationships and patterns in data, making them well-suited for stock forecasting tasks. However, they do not have memory, which limits their ability to handle time series data.
- Recurrent neural networks (RNNs): RNNs are a type of neural network specifically designed to process sequential data, such as time series or natural language. Unlike feedforward networks, RNNs have loops that allow them to maintain a hidden state, effectively “remembering” past information. This memory feature makes RNNs suitable for stock forecasting, as they can capture trends and patterns in historical price data.
- Long short-term memory (LSTM) networks: LSTMs are a special type of RNN that can learn long-term dependencies in time series data, overcoming the “vanishing gradient” problem that hampers traditional RNNs. LSTMs have been widely used for stock forecasting due to their ability to capture both short-term and long-term patterns in price movements.
Deep learning techniques
- Convolutional neural networks (CNNs): CNNs are a type of deep learning algorithm primarily used for image recognition but can also be applied to stock forecasting. By using convolutional layers, CNNs can automatically learn features from the input data, such as patterns in stock price movements, and use them to make predictions. This technique can be especially useful for analyzing high-dimensional data, such as financial time series with multiple variables.
- Autoencoders: Autoencoders are a type of unsupervised deep learning algorithm that learns to encode and decode data by minimizing the reconstruction error. In stock forecasting, autoencoders can be used to compress high-dimensional financial data into a lower-dimensional representation, capturing essential features and patterns that can be fed into other algorithms for prediction.
- Reinforcement learning: Reinforcement learning is a type of machine learning where an agent learns to make decisions by interacting with an environment and receiving feedback in the form of rewards or penalties. In stock forecasting, reinforcement learning can be used to develop trading strategies, as the agent learns to buy, sell, or hold stocks based on the potential for profit and minimizing losses.
Benefits of AI-based stock forecasting for investors
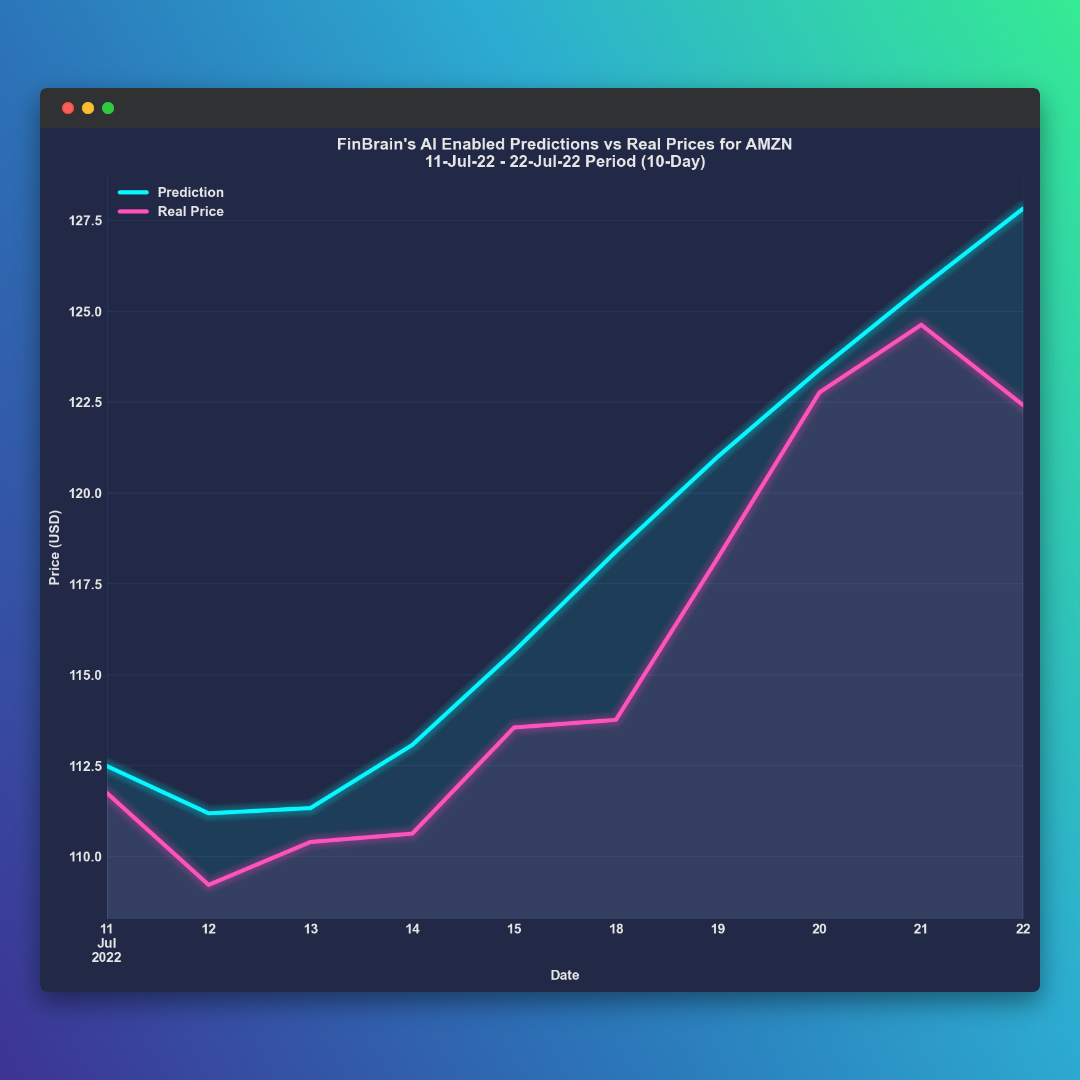
AI stock forecasting offers numerous benefits to investors, some of which are detailed below:
Improved accuracy and efficiency
AI algorithms can analyze vast amounts of data and identify patterns that may be difficult for humans to detect. As a result, AI-based stock forecasting can often provide more accurate predictions, helping investors make better-informed decisions.
Handling large volumes of data
AI algorithms can process and analyze massive amounts of data in a fraction of the time it would take a human analyst. This ability allows AI stock forecasting to consider multiple factors, such as historical data, financial reports, and market trends, simultaneously and quickly, leading to more comprehensive predictions.
Better risk management
AI stock forecasting can help investors identify potential risks and opportunities more effectively. By analyzing a wide range of data points, AI algorithms can detect potential market shifts and trends early, allowing investors to adjust their portfolios and manage risk proactively.
Personalized investment strategies
AI-based stock forecasting tools can offer personalized investment strategies based on an individual’s risk tolerance, investment goals, and preferences. This level of customization enables investors to create strategies tailored to their specific needs and objectives, potentially increasing their chances of success.
Real-time analysis and decision making
AI stock forecasting can provide real-time insights into market trends and stock price movements, allowing investors to react promptly to new information. This speed of analysis can be especially beneficial in fast-moving or volatile markets, where quick decision-making can be crucial to capitalize on opportunities or mitigate potential losses.
Reduction in human errors and biases
Human analysts can be prone to errors and biases that may affect their stock forecasts. AI algorithms, on the other hand, can help minimize these issues by objectively analyzing data and identifying patterns without being influenced by emotions or preconceived notions.
By incorporating AI stock forecasts provided by companies like FinBrain Technologies into their investment strategies, investors can leverage the numerous benefits of AI-based stock forecasting to make more informed decisions and potentially achieve higher returns.
By using AI stock forecasts provided by companies like FinBrain Technologies, investors can access alternative financial data such as News Sentiment Analysis, Mobile App Score Ratings, US Congress Members’ trades, Option Put-Call rations, and more. This data-driven investing approach can help individual investors maximize their investment returns by making more informed decisions based on AI-assisted analysis.
Challenges and limitations of AI in stock forecasting
Despite its potential, AI stock forecasting has its challenges and limitations:
- Overfitting and model complexity
- Data quality and preprocessing
- Lack of transparency and explainability
- Ethical concerns and potential manipulation
- Legal and regulatory issues
It’s essential for investors to be aware of these challenges and understand that AI stock forecasting is not infallible. However, when used correctly and responsibly, it can be a powerful tool for making more informed investment decisions.
Successful AI stock forecasting tools and platforms
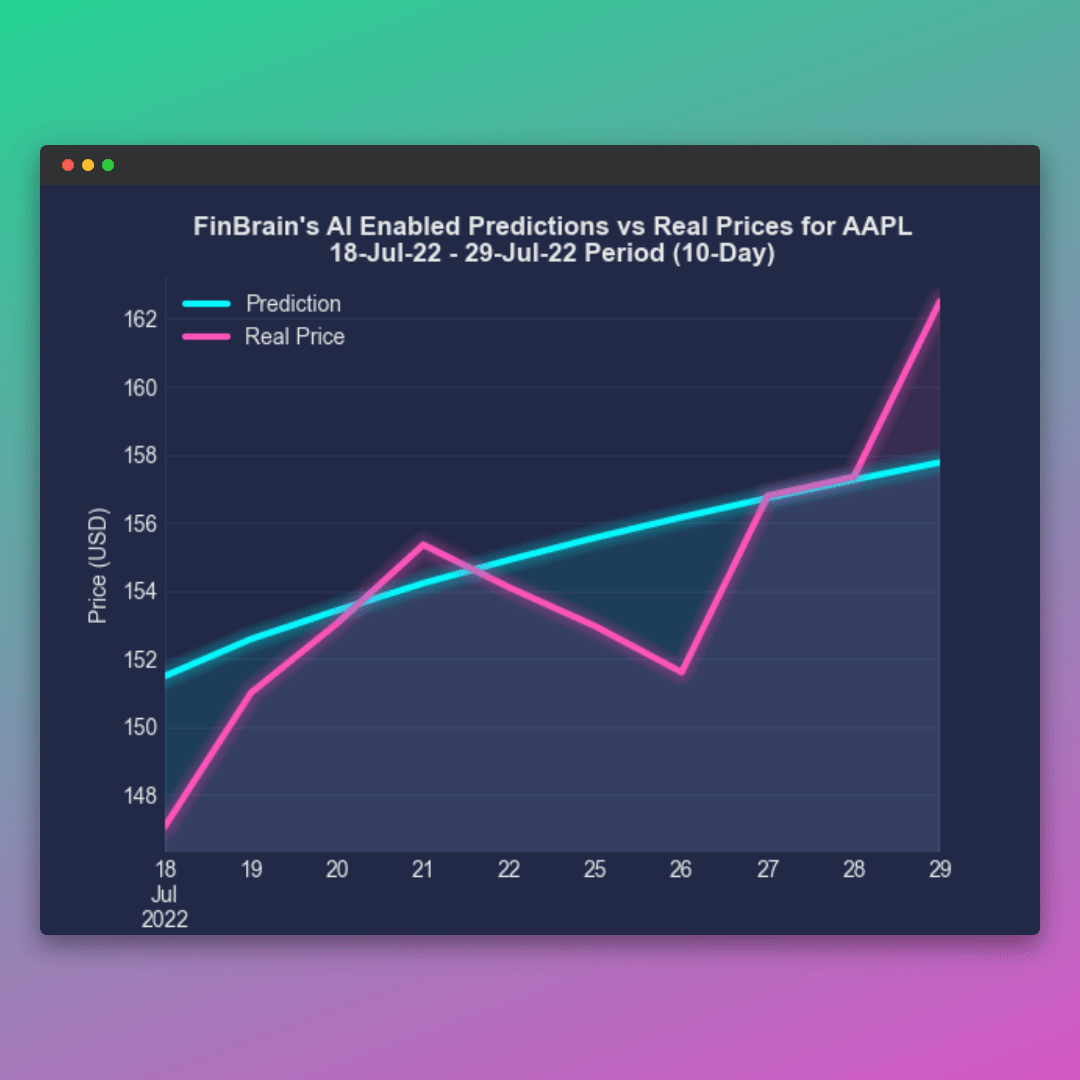
FinBrain Technologies is a prime example of a successful AI stock forecasting platform, providing AI stock forecasts for US & World stocks. Their FinBrain Terminal allows traders and investors from around the world to access AI stock forecasts and alternative data in their investment decisions. Additionally, FinBrain API enables institutional traders and data-driven funds to leverage the power of their massive amounts of data.
Several successful examples of AI stock forecasts by FinBrain Technologies can be found in these blog posts:
- Remarkable Forecast Accuracy for TSLA Stock
- Most Accurate Way to Forecast S&P 500 Stocks
- Highly Accurate AAPL Stock Price Forecast
- FinBrain as the Best Stock Prediction Website: AMZN Forecast Performance
These examples demonstrate the power of AI stock forecasting and its potential to help investors make more informed decisions.
Moreover, FinBrain’s Most Mentioned Tickers on WallStreetBets tracker is a valuable resource for investors, providing real-time information on the most discussed stocks in the popular WallStreetBets community.
Conclusion
AI stock forecasting has the potential to revolutionize the way investors approach the stock market by offering improved accuracy, efficiency, and the ability to process massive amounts of data. Companies like FinBrain Technologies are at the forefront of this transformation, providing AI stock forecasts and alternative financial data to help individual investors maximize their investment returns.
However, it’s crucial to understand that AI stock forecasting is not without its challenges and limitations. Investors must use AI-based tools responsibly and in conjunction with their knowledge and experience to make the best possible decisions. By integrating AI stock forecasting into their decision-making process, investors can gain a competitive edge and potentially achieve higher returns in the market.
FinBrain Technologies
Twitter • LinkedIn • Instagram • Facebook
99 Wall St. Suite #2023, New York, NY 10005
Leave a Reply